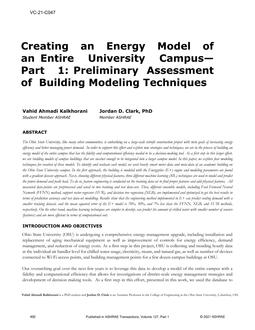
VC-21-C047 — Creating an Energy Model of an Entire University Campus¿Part 1: Preliminary Assessment of Building Modeling Techniques
- Comments Off on VC-21-C047 — Creating an Energy Model of an Entire University Campus¿Part 1: Preliminary Assessment of Building Modeling Techniques
- ASHRAE
Click here to purchase
The Ohio State University, like many other communities, is embarking on a large-scale retrofit construction project with twin goals of increasing energy efficiency and better managing power demand. In order to support this effort and explore new strategies and techniques, we are in the process of building an energy model of the entire campus that has the fidelity and computational efficiency needed to be a decision-making tool. As a first step in this larger effort, we are building models of campus buildings that are succinct enough to be integrated into a larger campus model. In this paper, we explore four modeling techniques for creation of these models. To identify and evaluate each model, we used hourly smart meter data and meta-data of an academic building on the Ohio State University campus. In the first approach, the building is modeled with the Energyplus (E+) engine and modeling parameters are found with a gradient descent approach. Next, choosing different physical features, three different machine learning (ML) techniques are used to model and predict the power demand and peak load. To do so, feature engineering is conducted on the training data-set to find proper features and add physical features. All measured data-points are preprocessed and saved in two training and test data-sets. Then, different ensemble models, including Feed Forward Neural Network (FFNN) method, support vector regressor (SVR), and decision tree regression (XGB), are implemented and optimized to get the best results in terms of prediction accuracy and test data-set modelling. Results show that the engineering method implemented in E+ can predict cooling demand with a smaller training dataset, and the mean squared error of the E+ model is 70%, 50%, and 7% less than the FFNN, XGB, and SVM methods, respectively. On the other hand, machine learning techniques are simpler to develop, can predict the amount of chilled water with smaller number of sensors (features) and are more efficient in terms of computational cost.
Citation: 2021 Virtual Conference Papers
Product Details
- Published:
- 2021
- Number of Pages:
- 9
- Units of Measure:
- Dual
- File Size:
- 1 file , 2.1 MB
- Product Code(s):
- D-VC-21-C047