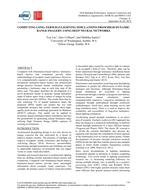
Computing Long-term Daylighting Simulations from High Dynamic Range Imagery Using Deep Neural Networks
- Comments Off on Computing Long-term Daylighting Simulations from High Dynamic Range Imagery Using Deep Neural Networks
- ASHRAE
Click here to purchase
Compared with illuminance-based metrics, luminance-based metrics and evaluations provide better understandings of occupant visual experience. However, it is computationally expensive and time consuming to incorporate luminance-based metrics into architectural design practice because annual simulations require generating a luminance map at each time step of the entire year. This paper describes the development of a novel prediction model to generate annual luminance maps of indoor space from a subset of images by using deep neural networks (DNNs). The results show that by only rendering 5% of annual luminance maps, the proposed DNNs model can predict the rest with comparable accuracy that closely matches those high-quality point-in-time renderings generated by Radiance (RPICT) software. This model can be applied to accelerate annual luminance-based simulations and lays the groundwork for generating annual luminance maps utilizing High Dynamic Range (HDR) captures of existing environments.
Citation: ASHRAE/IBPSA-USA Bldg Simulation Conf, Sept 2018
Product Details
- Published:
- 2018
- Number of Pages:
- 8
- Units of Measure:
- Dual
- File Size:
- 1 file , 3.3 MB
- Product Code(s):
- D-BSC18-C018