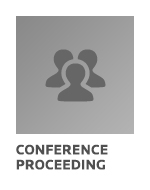
C081 — Generation of Load Profile Based on GAN and LSTM Network in Smart Grid
- Comments Off on C081 — Generation of Load Profile Based on GAN and LSTM Network in Smart Grid
- ASHRAE
Click here to purchase
With the development of artificial intelligence technology in recent years, more and more researchers apply these technologies to the related research of smart grid. However, machine learning models are essentially data-driven models, which are needed to be trained on a great amount of data, and then they are able to predict and analyze new data. However, in the process of acquiring smart meter data through transmission system, there are many problems, such as time consuming, high smart meter deployment cost, and data privacy concern. These problems make it difficult to obtain load data, which hinders the research and application of data-driven methods in the smart grid field. Therefore, generating load profiles is a promising solution. In this study, we propose Long Short Term Memory Generative Adversarial Network (LSTMGAN) model to generate load profile. GAN module is used to generate load profiles. The trained LSTM prediction network serves as supervision module. It considers the difference between latent features of generated load profile and real load profile as feature loss. The latent series representation has been improved by adding the feature loss to the original generator loss. The experiment is conducted on load profile of office building and commercial building in Shenzhen. We evaluate the proposed model from similarity, variability and diversity. The result shows that LSTMGAN model can generate load profiles which can reflect not only the real load trend but also stochastic behavior and perform better than traditional GAN and VAE in load profile generation. Keywords—Generative Adversarial Network, Long Short Term Memory, load profile generation, data-driven
Product Details
- Published:
- 2023
- Number of Pages:
- 8
- Units of Measure:
- Dual
- File Size:
- 1 file , 1.9 MB
- Product Code(s):
- D-AT-23-C081
- Note:
- This product is unavailable in Russia, Belarus