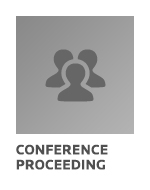
C079 — Short-Term Occupant Numbering Prediction via Machine Learning Approaches
- Comments Off on C079 — Short-Term Occupant Numbering Prediction via Machine Learning Approaches
- ASHRAE
Click here to purchase
Occupancy behavior plays an essential part in smart building operation. Developing an appropriate algorithm to predict occupancy information will bring a better control for Heating Ventilation & Air Conditioning system, and indoor health. However, due to the strong stochasticity of occupancy behavior, it is much harder to predict occupant count than occupant state. There is a lot of studies working on occupancy presence or arrive-departure time prediction, only a few researchers focus on the occupant count prediction. The lack of occupant count prediction limits the development of demand-controlled ventilation. In this study, 1) A set of ground truth data was collected via state-of-the-art people counting sensor. 2) A flatten preprocessing method was used to smooth the collected data of occupant number. 3) Seven different models (ARMA_ANN model, RNN model, LSTM model, Nonhomogeneous Markov with change point detection model, XGBoost model, Random Forest model and ANN_Range model) were used to predict the room occupant count from 15 minutes to 24 hours ahead. We found that XGBoost model, Random Forest model and ARMA_ANN model have similar performance and they all outperforms than the other models by a 3% to 13% mismatch rate reduction and reduce the computation time. Each model could predict the number of occupants with 85% accuracy with one-person offset and the accuracy for 15 minutes ahead prediction could reach 95% with one-person offset. LSTM model works slightly better than RNN model and both of them had a smoother prediction. None of these seven models could track the abrupt changes.
Product Details
- Published:
- 2023
- Number of Pages:
- 9
- Units of Measure:
- Dual
- File Size:
- 1 file , 2.2 MB
- Product Code(s):
- D-AT-23-C079
- Note:
- This product is unavailable in Russia, Belarus