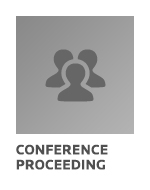
C022 — Data-Driven Machine Learning Model Performance of Real Annual Natural Gas Consumption in Residential Buildings
- Comments Off on C022 — Data-Driven Machine Learning Model Performance of Real Annual Natural Gas Consumption in Residential Buildings
- ASHRAE
To achieve climate neutrality by 2050, Building-Stock Energy Models (BSEMs) are key tools in comparing competing building energy reduction strategies. Yet, at present, existing regulatory energy performance calculation methods poorly estimate the real building energy use and widely overestimates the potential energy savings. Promising data-driven machine learning models, such as gradient boosting machines and support vector machines are gaining considerable traction in a wide range of applications. In this paper, we will evaluate the performance of common data-driven blackbox models and evaluate whether they could potentially replace the present regulatory calculation method for prediction and/or policy making.
Product Details
- Published:
- 2022
- Number of Pages:
- 8
- Units of Measure:
- Dual
- File Size:
- 1 file , 8.5 MB
- Product Code(s):
- D-BCS22-C022
- Note:
- This product is unavailable in Russia, Belarus