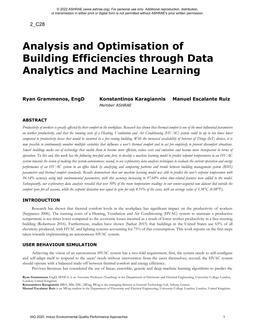
Analysis and Optimisation of Building Efficiencies through Data Analytics and Machine Learning
- Comments Off on Analysis and Optimisation of Building Efficiencies through Data Analytics and Machine Learning
- ASHRAE
Click here to purchase
Productivity of workers is greatly affected by their comfort in the workplace. Research has shown that thermal comfort is one of the most influential parameterson worker productivity, and that the running costs of a Heating, Ventilation and Air Conditioning (HVAC) system could be up to ten times lower compared to productivity losses that would be incurred in a free-runing building. With the increased availability of Internet of Things (IoT) devices, it is now possible to continuously monitor multiple variables that influence a user’s thermal comfort and to act pre-emptively to prevent discomfort situations. Smart buildings make use of technology that enable them to become more efficient, reduce costs and emissions and become more transparent in terms of operation. To this end, this work has the following two-fold aim; first, to develop a machine learning model to predict setpoint temperatures in an HVAC system towards the vision of making this system autonomous; second, to use exploratory data analysis techniques to evaluate the current operation and energy performance of an HVAC system in an office block by analyzing and comparing patterns and trends between building management system (BMS)parameters and thermal comfort standards. Results demonstrate that our machine learning model was able to predict the user’s setpoint temperature with 94.14% accuracy using only environmental parameters, with this accuracy increasing to 97.68% when time-related features were added to the model. Subsequently, our exploratory data analysis revealed that over 50% of the room temperature readings in our sensor-acquired raw dataset lied outside the comfort zone for all seasons, while the setpoint deviation was equal to zero for only 4.31% of the cases, with an average value of 3.38°C (6.08°F).
Product Details
- Published:
- 2022
- Number of Pages:
- 8
- Units of Measure:
- Dual
- File Size:
- 1 file , 1.1 MB
- Product Code(s):
- D-IAQ2020-C2-C28
- Note:
- This product is unavailable in Russia, Belarus