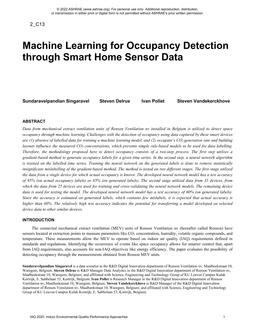
Machine Learning for Occupancy Detection through Smart Home Sensor Data
- Comments Off on Machine Learning for Occupancy Detection through Smart Home Sensor Data
- ASHRAE
Click here to purchase
Data from mechanical extract ventilation units of Renson Ventilation nv installed in Belgium is utilized to detect space occupancy through machine learning. Challenges with the detection of occupancy using data captured by these smart devicesare (1) absence of labelled data for training a machine learning model, and (2) occupant’s CO2 generation rate and building layouts influence the measured CO2 concentrations, which prevents simple rule-based models to be used for data labelling. Therefore, the methodology proposed here to detect occupancy consists of a two-step process. The first step utilizes a gradient-based method to generate occupancy labels for a given time series. In the second step, a neural network algorithm is trained on the labelled time series. Training the neural network on the generated labels is done to remove statistically insignificant mislabelling of the gradient-based method. The method is tested on two different stages. The first stage utilizedthe data from a single device for which actual occupancy is known. The developed neural network model has a test accuracy of 95% (on actual occupancy labels) or 85% (on generated labels). The second stage utilized data from 35 devices, fromwhich the data from 25 devices are used for training and cross-validating the neural network models. The remaining device data is used for testing the model. The developed neural network model has a test accuracy of 60% (on generated labels).Since the accuracy is estimated on generated labels, which contains few mislabels, it is expected that actual accuracy is higher than 60%. The relatively high test accuracy indicates the potential for transferring a model developed on selected device data to other similar devices.
Product Details
- Published:
- 2022
- Number of Pages:
- 8
- File Size:
- 1 file , 1.1 MB
- Product Code(s):
- D-IAQ2020-C2-C13
- Note:
- This product is unavailable in Russia, Belarus